
Nuclear and Particle Physics- using liquid drop nuclear mass formulaNuclear and Particle PhysicsA typical binding energy for an alpha particle in a heavy nucleus is about 28.3 MeV. Using the liquid drop nuclear mass formula, determine for what nuclear mass number alpha decay is energetically allowed. Ignore the spin pairing term. Assume that for heavy nuclei, Z≈A/2.25.Hint: Parametrize the change in binding energy of the heavy nuclei as (screenshot of equation was uploaded)SAMPLE-Exam A ETF5952 Questions for Final Exam INSTRUCTIONS TO STUDENTS 1. Answer ALL questions and type all your answers. Any answers by image or handwriting will received zero mark. 2. When certain decimal places for answers are stated, your answer should be rounded. 3. When word limits for answers are stated, your answers should satisfy the limits. If your answer exceeds the stated limit, you will receive zero mark for the question. 1 Question 1 (25 points=5+5+5+10) We consider a data set on weekly stock return. The variables in the data set are as follows: • Year: The year that the observation was recorded • Lag1: Percentage return for previous week • Lag2: Percentage return for 2 weeks previous • Lag3: Percentage return for 3 weeks previous • Lag4: Percentage return for 4 weeks previous • Lag5: Percentage return for 5 weeks previous • Volume: Volume of shares traded (average number of daily shares traded in billions) • Today: Percentage return for this week • Direction: A factor with levels Down and Up indicating whether the market had a positive or negative return on a given week 1. We obtain the sample statistics. Answer the average weekly return of this week’s variable. Year Lag1 Lag2 Lag3 Lag4 Lag5 1996 : 53 Min. :-18.1950 Min. :-18.1950 Min. :-18.1950 Min. :-18.1950 Min. :-18.1950 2007 : 53 1st Qu.: -1.1540 1st Qu.: -1.1540 1st Qu.: -1.1580 1st Qu.: -1.1580 1st Qu.: -1.1660 1991 : 52 Median : 0.2410 Median : 0.2410 Median : 0.2410 Median : 0.2380 Median : 0.2340 1992 : 52 Mean : 0.1506 Mean : 0.1511 Mean : 0.1472 Mean : 0.1458 Mean : 0.1399 1993 : 52 3rd Qu.: 1.4050 3rd Qu.: 1.4090 3rd Qu.: 1.4090 3rd Qu.: 1.4090 3rd Qu.: 1.4050 1994 : 52 Max. : 12.0260 Max. : 12.0260 Max. : 12.0260 Max. : 12.0260 Max. : 12.0260 (Other):775 Volume Today Direction time Min. :0.08747 Min. :-18.1950 Down:484 Min. : 1 1st Qu.:0.33202 1st Qu.: -1.1540 Up :605 1st Qu.: 273 Median :1.00268 Median : 0.2410 Median : 545 Mean :1.57462 Mean : 0.1499 Mean : 545 3rd Qu.:2.05373 3rd Qu.: 1.4050 3rd Qu.: 817 Max. :9.32821 Max. : 12.0260 Max. :1089 2. We estimate an autoregressitve with lag order of 1 or AR(1) model as reported below. Explain the effect of Lag1 on Today. glm(formula = Today ~ Lag1, data = DATA) Deviance Residuals: Min 1Q Median 3Q Max -19.061 -1.271 0.113 1.280 11.235 Coefficients: Estimate Std. Error t value Pr(>|t|) (Intercept) 0.16120 0.07140 2.258 0.0242 * Lag1 -0.07503 0.03024 -2.481 0.0133 * — Signif. codes: 0 *** 0.001 ** 0.01 * 0.05 . 0.1 1 3. We estimate an autoregressitve with lag order of 5 or AR(5) model as reported below. Explain the effect of Volume on Today (no more than 20 words). 2 glm(formula = Today ~ Lag1 + Lag2 + Lag3 + Lag4 + Lag5 + Volume, data = DATA) Deviance Residuals: Min 1Q Median 3Q Max -18.5410 -1.2622 0.0873 1.2579 11.9316 Coefficients: Estimate Std. Error t value Pr(>|t|) (Intercept) 0.25050 0.09964 2.514 0.0121 * Lag1 -0.07143 0.03046 -2.346 0.0192 * Lag2 0.04760 0.03058 1.556 0.1199 Lag3 -0.06857 0.03045 -2.252 0.0245 * Lag4 -0.02215 0.03047 -0.727 0.4674 Lag5 0.01406 0.03039 0.463 0.6437 Volume -0.05441 0.04274 -1.273 0.2033 — Signif. codes: 0 *** 0.001 ** 0.01 * 0.05 . 0.1 1 4. We estimate the model with more variables and also obtain the AIC for all three models, as reported below (fit1, fit2 and fit3 are estimation results from Question1.2, Question1.3, Question1.4, respectively). In terms of R2 , the model here is better. Explain why this model is better or worse for predicting stock return. (no more than 30 words). glm(formula = Today ~ Lag1 + Lag2 + Lag3 + Lag4 + Lag5 + Volume + Year, data = DATA) Deviance Residuals: Min 1Q Median 3Q Max -18.0478 -1.2246 0.0719 1.2435 12.4662 Coefficients: Estimate Std. Error t value Pr(>|t|) (Intercept) -0.05787 0.34205 -0.169 0.86569 Lag1 -0.09849 0.03104 -3.173 0.00155 ** Lag2 0.02080 0.03149 0.661 0.50907 Lag3 -0.09284 0.03101 -2.994 0.00281 ** Lag4 -0.04905 0.03100 -1.582 0.11394 Lag5 -0.01007 0.03080 -0.327 0.74389 Volume -0.06976 0.15874 -0.439 0.66043 Year1991 0.68642 0.47174 1.455 0.14594 Year1992 0.20143 0.47144 0.427 0.66926 Year1993 0.24403 0.47139 0.518 0.60478 Year1994 0.04760 0.47122 0.101 0.91956 Year1995 0.78668 0.47503 1.656 0.09800 . Year1996 0.55581 0.47238 1.177 0.23961 Year1997 0.74433 0.47768 1.558 0.11948 Year1998 0.69017 0.48118 1.434 0.15177 Year1999 0.58028 0.48512 1.196 0.23190 Year2000 -0.04876 0.49021 -0.099 0.92079 Year2001 -0.09390 0.49905 -0.188 0.85078 Year2002 -0.39523 0.50943 -0.776 0.43803 Year2003 0.64860 0.51383 1.262 0.20712 Year2004 0.38829 0.51445 0.755 0.45056 Year2005 0.28258 0.54832 0.515 0.60641 Year2006 0.52796 0.59237 0.891 0.37299 3 Year2007 0.31644 0.67343 0.470 0.63853 Year2008 -0.48258 0.88789 -0.544 0.58689 Year2009 0.97455 0.99170 0.983 0.32598 Year2010 0.69020 0.84774 0.814 0.41573 — Signif. codes: 0 *** 0.001 ** 0.01 * 0.05 . 0.1 1 > c(AIC(fit1), AIC(fit2), AIC(fit3) ) [1] 4956.627 4956.663 4969.627 4 Question 2 (25 points=5+5+5+10) We consider a data set on credit card default. The variables in the data set are • default: A factor with levels No and Yes indicating whether the customer defaulted on their debt • student: A factor with levels No and Yes indicating whether the customer is a student • balance: The average balance that the customer has remaining on their credit card after making their monthly payment • income: Income of customer 1. We obtain summary statistics and a frequency table as below. Obtain the probability of default = YES and Student = NO and the probability of default = YES condition on student = NO (2 decimal places). > summary(DATA) default student balance income No :9667 No :7056 Min. : 0.0 Min. : 772 Yes: 333 Yes:2944 1st Qu.: 481.7 1st Qu.:21340 Median : 823.6 Median :34553 Mean : 835.4 Mean :33517 3rd Qu.:1166.3 3rd Qu.:43808 Max. :2654.3 Max. :73554 > table(DATA$default, DATA$student) No Yes No 6850 2817 Yes 206 127 2. We obtain an estimate result as below. Interpret the estimated coefficient of income. glm(formula = default ~ balance + income + student, family = “binomial”, data = DATA) Deviance Residuals: Min 1Q Median 3Q Max -2.4691 -0.1418 -0.0557 -0.0203 3.7383 Coefficients: Estimate Std. Error z value Pr(>|z|) (Intercept) -1.087e+01 4.923e-01 -22.080 < 2e-16 *** balance 5.737e-03 2.319e-04 24.738 < 2e-16 *** income 3.033e-06 8.203e-06 0.370 0.71152 studentYes -6.468e-01 2.363e-01 -2.738 0.00619 ** — Signif. codes: 0 *** 0.001 ** 0.01 * 0.05 . 0.1 1 3. Using the previous estimation result, interpret the estimated coefficient of student. 4. We obtain another estimate result as below. Interpret the effect of balance in the result (no more than 30 words, 4 decimal places). glm(formula = default ~ balance * student + income, family = “binomial”, data = DATA) Deviance Residuals: Min 1Q Median 3Q Max -2.4949 -0.1417 -0.0555 -0.0202 3.7576 5 Coefficients: Estimate Std. Error z value Pr(>|z|) (Intercept) -1.099e+01 5.667e-01 -19.399 <2e-16 *** balance 5.817e-03 2.938e-04 19.801 <2e-16 *** studentYes -2.856e-01 8.239e-01 -0.347 0.729 income 3.016e-06 8.226e-06 0.367 0.714 balance:studentYes -2.184e-04 4.781e-04 -0.457 0.648 — Signif. codes: 0 *** 0.001 ** 0.01 * 0.05 . 0.1 1 6 Question 3 (25 points=5+5+5+10) We consider a data set on cars. The data set includes the following variables: • mpg: miles per gallon • cylinders: Number of cylinders between 4 and 8 • displacement: Engine displacement (cu. inches) • horsepower: Engine horsepower • weight: Vehicle weight (lbs.) • acceleration: Time to accelerate from 0 to 60 mph (sec.) • year: Model year (modulo 100) • origin: Origin of car (1. American, 2. European, 3. Japanese) • name: Vehicle name The summary statistics is as follows: mpg cylinders displacement horsepower weight acceleration year Min. : 9.00 Min. :3.000 Min. : 68.0 Min. : 46.0 Min. :1613 Min. : 8.00 73 : 40 1st Qu.:17.00 1st Qu.:4.000 1st Qu.:105.0 1st Qu.: 75.0 1st Qu.:2225 1st Qu.:13.78 78 : 36 Median :22.75 Median :4.000 Median :151.0 Median : 93.5 Median :2804 Median :15.50 76 : 34 Mean :23.45 Mean :5.472 Mean :194.4 Mean :104.5 Mean :2978 Mean :15.54 75 : 30 3rd Qu.:29.00 3rd Qu.:8.000 3rd Qu.:275.8 3rd Qu.:126.0 3rd Qu.:3615 3rd Qu.:17.02 82 : 30 Max. :46.60 Max. :8.000 Max. :455.0 Max. :230.0 Max. :5140 Max. :24.80 70 : 29 (Other):193 origin name Min. :1.000 amc matador : 5 1st Qu.:1.000 ford pinto : 5 Median :1.000 toyota corolla : 5 Mean :1.577 amc gremlin : 4 3rd Qu.:2.000 amc hornet : 4 Max. :3.000 chevrolet chevette: 4 (Other) :365 1. We obtain a regression result as below. Explain the effect of weight on miles per gallon (no more than 30 words.) glm(formula = log(mpg) ~ log(weight) + cylinders + displacement + horsepower + acceleration + year, data = DATA) Deviance Residuals: Min 1Q Median 3Q Max -0.34100 -0.05913 0.00180 0.05995 0.37563 Coefficients: Estimate Std. Error t value Pr(>|t|) (Intercept) 1.133e+01 1.097e+00 10.334 < 2e-16 *** log(weight) -1.056e+00 1.560e-01 -6.772 4.96e-11 *** cylinders -2.045e-02 1.062e-02 -1.924 0.055082 . displacement 1.166e-04 2.351e-04 0.496 0.620209 horsepower -1.656e-03 4.706e-04 -3.520 0.000485 *** acceleration -3.262e-03 3.236e-03 -1.008 0.314127 year71 1.542e-03 3.095e-02 0.050 0.960290 year72 -2.846e-02 2.996e-02 -0.950 0.342860 year73 -5.343e-02 2.721e-02 -1.964 0.050319 . year74 3.420e-02 3.222e-02 1.061 0.289294 year75 3.843e-02 3.131e-02 1.227 0.220567 7 year76 6.273e-02 3.010e-02 2.084 0.037830 * year77 1.163e-01 3.067e-02 3.791 0.000175 *** year78 1.316e-01 2.903e-02 4.533 7.84e-06 *** year79 2.196e-01 3.072e-02 7.148 4.67e-12 *** year80 3.328e-01 3.238e-02 10.280 < 2e-16 *** year81 2.518e-01 3.186e-02 7.904 3.07e-14 *** year82 2.926e-01 3.105e-02 9.423 < 2e-16 *** — Signif. codes: 0 *** 0.001 ** 0.01 * 0.05 . 0.1 1 2. We apply the lasso procedure, where the dependent variables is log of mpg and the rest of variables in the data are used as regressors. Explain why we should not simply run standard regression. > x = sparse.model.matrix(log(mpg) ~ ., data=DATA)[,-1] > y = log(DATA$mpg) > dim(x) [1] 392 321 > x = sparse.model.matrix(log(mpg) ~ ., data=DATA)[,-1] > x = sparse.model.matrix(log(mpg) ~ ., data=DATA)[,-1] > y = log(DATA$mpg) > dim(x) [1] 392 321 > sclasso = gamlr(x, y, family = “gaussian”, nfold=10) > sum(coef(sclasso) !=0) [1] 58 3. In outputs from the lasso above, explain whether the lasso works properly (no more than 20 words). 4. We obtain a plot after we implement the lasso procedure above. This plot shows trajectories of estimated coefficients. Explain why lines spread widely on the left side and does not on the right side (no more than 40 words). 8 Question 4 (25 points=5+5+5+10) We have a data set collected from 506 geographical areas and the data set contains a list of variables: • price: median housing price in US$1,000, • crime: crimes committed per capita • nox: nitric oxide pollution per 100m • rooms: average number of rooms • dist: weighted distance to city center • radial: access index to radial highways (radial), • stratio: average student-teacher ratio We estimate a regression tree by using price as the dependent variable and the other variables as regressors. The estimation result is reported in Figure 1. Figure 1: Regression Tree Result 1. The far-right node has 45 and 6%. Explain those numbers (no more than 20 words.) 2. Interpret prediction from the above estimation result for an area with rooms = 4, stratio = 15, dist = 2, crime = 8 and nox = 3 (no more than 20 words). 3. Find the node with 18 and 8% and explain characters of observations in the node. 4. Explain what possibly causes low housing prices at the far-left terminal node of the regression tree result. 9
Get professional assignment help cheaply
Are you busy and do not have time to handle your assignment? Are you scared that your paper will not make the grade? Do you have responsibilities that may hinder you from turning in your assignment on time? Are you tired and can barely handle your assignment? Are your grades inconsistent?
Whichever your reason may is, it is valid! You can get professional academic help from our service at affordable rates. We have a team of professional academic writers who can handle all your assignments.
Our essay writers are graduates with diplomas, bachelor, masters, Ph.D., and doctorate degrees in various subjects. The minimum requirement to be an essay writer with our essay writing service is to have a college diploma. When assigning your order, we match the paper subject with the area of specialization of the writer.
Why choose our academic writing service?
Plagiarism free papers
Timely delivery
Any deadline
Skilled, Experienced Native English Writers
Subject-relevant academic writer
Adherence to paper instructions
Ability to tackle bulk assignments
Reasonable prices
24/7 Customer Support
Get superb grades consistently
Get Professional Assignment Help Cheaply
Are you busy and do not have time to handle your assignment? Are you scared that your paper will not make the grade? Do you have responsibilities that may hinder you from turning in your assignment on time? Are you tired and can barely handle your assignment? Are your grades inconsistent?
Whichever your reason may is, it is valid! You can get professional academic help from our service at affordable rates. We have a team of professional academic writers who can handle all your assignments.
Our essay writers are graduates with diplomas, bachelor’s, masters, Ph.D., and doctorate degrees in various subjects. The minimum requirement to be an essay writer with our essay writing service is to have a college diploma. When assigning your order, we match the paper subject with the area of specialization of the writer.
Why Choose Our Academic Writing Service?
Plagiarism free papers
Timely delivery
Any deadline
Skilled, Experienced Native English Writers
Subject-relevant academic writer
Adherence to paper instructions
Ability to tackle bulk assignments
Reasonable prices
24/7 Customer Support
Get superb grades consistently
How It Works
1. Place an order
You fill all the paper instructions in the order form. Make sure you include all the helpful materials so that our academic writers can deliver the perfect paper. It will also help to eliminate unnecessary revisions.
2. Pay for the order
Proceed to pay for the paper so that it can be assigned to one of our expert academic writers. The paper subject is matched with the writer’s area of specialization.
3. Track the progress
You communicate with the writer and know about the progress of the paper. The client can ask the writer for drafts of the paper. The client can upload extra material and include additional instructions from the lecturer. Receive a paper.
4. Download the paper
The paper is sent to your email and uploaded to your personal account. You also get a plagiarism report attached to your paper.
PLACE THIS ORDER OR A SIMILAR ORDER WITH Essay fount TODAY AND GET AN AMAZING DISCOUNT
The post Exam instructions for physician practicals appeared first on Essay fount.
What Students Are Saying About Us
.......... Customer ID: 12*** | Rating: ⭐⭐⭐⭐⭐"Honestly, I was afraid to send my paper to you, but you proved you are a trustworthy service. My essay was done in less than a day, and I received a brilliant piece. I didn’t even believe it was my essay at first 🙂 Great job, thank you!"
.......... Customer ID: 11***| Rating: ⭐⭐⭐⭐⭐
"This company is the best there is. They saved me so many times, I cannot even keep count. Now I recommend it to all my friends, and none of them have complained about it. The writers here are excellent."
"Order a custom Paper on Similar Assignment at essayfount.com! No Plagiarism! Enjoy 20% Discount!"
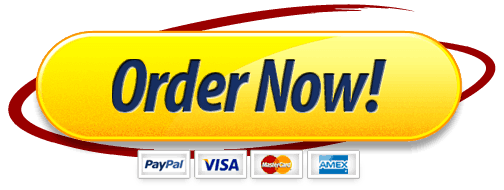